AI is Not a Wonder Tool that Substitutes Topology Optimization
Interview with With Prof. Ole Sigmund of DTU
Ole Sigmund is a Professor and Villum Investigator at the Department of Civil and Mechanical Engineering at the Technical University of Denmark. He is one of the founders and main contributor to the development of topology optimization methods in academia and industry having published more than 300 academic papers on the subject.
Following is an interview in advance of his presentation on ‘Perfect Design for Imperfect AM’ at CDFAM Berlin, 2024.
Advancements in AI and Topology Optimization?
In our last discussion in 2022, you mentioned that AI seemed to be a dead-end for topology optimization based on the findings in your co-authored paper “On the use of artificial neural networks in topology optimization.” Have there been any significant developments since then that have altered your perspective?
I still stand with our original conclusions.
AI interpolates based on data or observations and is bad at extrapolating.
In topology optimization we are searching for the extreme and at the same time we have through time developed extremely efficient algorithms to find the optimal structures. One also has to keep in mind that data is very expensive to generate.
In the paper we discussed break-even vs generality scores for evaluating AI-based approaches. The break-even score is the number of uses of the trained network before the training cost has been covered and the generality score measures the sensitivity to change of boundary conditions, geometries or other optimization settings.
For very specialized applications with simple boundary conditions that need to be run again and again (like e.g. lattice identification for customization of uni-directional force-displacement curves for shoe-soles), a high break-even and a low generality score may be tolerable. However, for general problems existing approaches are far superior.
It is also worth noting that AI is yet to be demonstrated as a tool capable of solving PDEs on arbitrary domains with arbitrary boundary conditions. This provides the basis for every topology optimization problem and hence it is, for now, optimistic to believe that AI can be used for inverse problems, when it cannot even solve the underlying physics reliably.
Could factors such as reduced computational costs, access to more data, or improved methodologies potentially make AI a viable tool for topology optimization?
I will again refer to the break-even and generality scores mentioned before. To train a network that is generally applicable in mechanical and multiphysics design would require immense resources.
That computers are getting faster or AI training methodologies getting better does not change the overall energy picture, nor does it overcome the conflict between the interpolative properties of current AI and the discontinuous solution-spaces in TO.
We can solve thousands of standard optimization problems with a guaranty of near optimal designs before the cost of the training of a network that may or may not provide feasible solutions has been covered.
In the above I am talking about the direct substitution of the topology optimization (TO) process by AI. There may, however, be repeated subtasks of the TO process with efficiency improvement potential as we reviewed in the paper. Still, even for this we are still waiting to see game changers.
In fact, we ourselves thought that we had found a case where we could train a dehomogenization network on artificial data and obtain good speedups.
Subsequently, however, my Pd.D.-student Rebekka Woldseth found an even more efficient approach based on procedural noise concepts and phasors that I will discuss in my presentation.
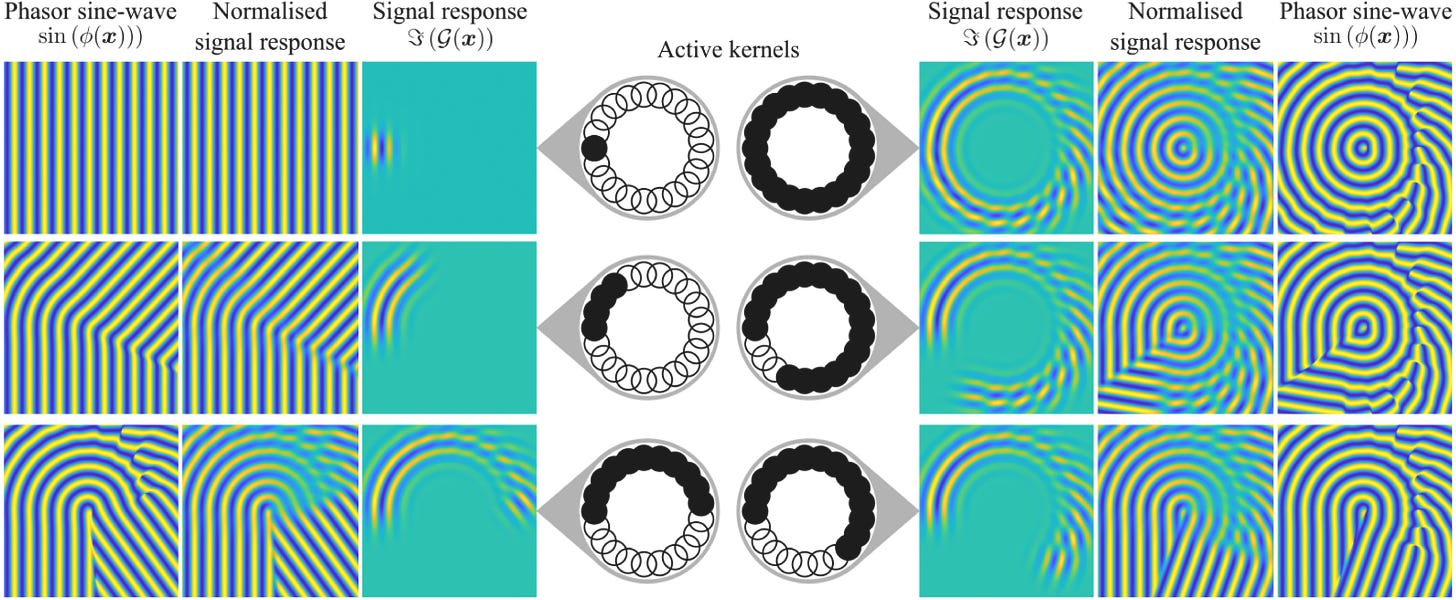
CDFAM Presentation Overview:
As you prepare for your presentation at CDFAM in Berlin, could you provide us with an overview of the topics you will be addressing? What are the key points and research that you plan to highlight during the event?
There are many things that I’d like to address but time is limited so I will probably concentrate on showcasing our works in dehomogenization, mention various pitfalls in lattice design and touch a bit on the AI aspects as well. Dehomogenization refers to multiscale topology optimization where we utilize the knowledge of optimal microstructures that are oriented and dimensioned according to local stresses.
This allows for extremely efficient computational procedures that only require coarse finite element discretizations followed by fine-scale realization of the optimized structures using projection-based computer graphics concepts. The obtained lattice structures provide extreme stiffness, but may be subject to local yield or buckling effects that we are now also trying to account for.
Verification of Complex Geometries
Once these complex lattice geometries have been created, what methods do you employ to verify their effectiveness and integrity? Do you see potential benefits in advances in implicit and/or meshless simulations, or is physical testing still predominantly required?
We have published works on verification of smaller dehomogenized structures. However, when it comes to optimizing a high-resolution airplane wing efficiently using multi-scale approaches, we are still challenged in visualizing the full structure, let alone discretize it for post-evaluation.
Advice and Expectations for Software Developers at CDFAM:
With many leading software developers of topology optimization, lattice design, and simulation tools attending CDFAM, what advice would you offer them? What developments or innovations do you hope to see presented at the event?
In order to simulate and verify complex multiscale structures we need robust and efficient algorithms and datastructures for converting point maps or implicit surfaces to high-resolution body-fitted FE-meshes suitable for accurate physics simulations.
Cross-Disciplinary Insights from Topology Optimization and Machine Learning:
How do you perceive the integration of topology optimization and machine learning in narrow fields such as nanophotonics lenses and optics influencing mechanical engineering approaches and applications in other specialized fields?
Apart from our mechanical engineering activities, we are developing TO approaches for many other physics problems.
Examples are acoustics, antennas, nano-photonics, quantum optomechanics, thermotics, etc.. TO is gaining popularity in all these fields. Specifically in nano-photonics, where light is guided on silicon chips by appropriate distributions of dielectrica and cavities, TO has become extremely popular.
Nevertheless, the applicability of AI in this area is also debatable and as in the mechanics field we often miss critical examination of new approaches in terms of break-even and generality scores.
In parallel to the shoe sole problem discussed above, a simple and repeated identification problem in nano-photonics, that may have some potential for use of AI, is index engineering and microstructure identification for meta-lenses.
Key Takeaways and Learning Goals at CDFAM:
For participants of the CDFAM Symposium, what key insights do you hope they will take away from your presentation, and are there specific areas of collaboration or knowledge exchange you are particularly interested in exploring during the event?
I hope to convince the audience that AI is not a wonder tool that substitutes topology optimization approaches but needs to be applied for the right (sub)tasks and with a critical mind. Also, I hope to open the audience’s eyes to pitfalls in the use of lattice structures for mechanical design.
Finally, I hope that my talk will inspire lively and inspiring discussions leading to further developments and paradigm shifts in the exciting field of inverse design, AM and topology optimization.
* Images from: Phasor noise for dehomogenisation in 2D multiscale topology optimisation. Authors: Rebekka V. Woldseth, J. Andreas Bærentzen, Ole Sigmund
** Image from Topology optimization of multi-scale structures: a review. Authors. Jun Wu, Ole Sigmund & Jeroen P. Groen
Join Ole and other experts in computational design, AI in engineering and advanced manufacturing at CDFAM Berlin, May 7-8, 2024