Optimization for DfAM & Uncertainty
Robot Gripper design with Rafinex's Möbius: Stochastic Topology Optimizer
Guest post by Armin Lohwasser, Chief Business Officer of Rafinex
In the large domain of optimization solutions, a newcomer sets out on a vastly different track to generate sustainable designs for Additive Manufacturing: Rafinex.
The start-up's core technology is leveraging stochastics to anticipate uncertainties during design optimization to generate truly robust designs. Accounting for these uncertainties is critical as they affect the structural performance of designs throughout their service life.
Robustness is paramount! This robustness supports DFAM in that the deviations from an idealized load-case, as well as the AM process related induced variabilities and the still prevailing inaccuracies are compensated by the structural robustness of the design.
The experts at Rafinex, working from all over Europe, have launched an optimizer called Möbius that promises to effectively anticipate and manage this uncertainty and to create parts that are fit for real-life service.
In a recent project, Rafinex joined forces with Nuform to demonstrate the effective adoption of the optimizer for an DFAM adoption.
Nuform is a consulting company working directly with their partners and customers on innovative activities or - as they coin it - "the extended workbench of development" with one focus on AM as it is a powerful enabler for many leap-frogging innovations.
The identified use case was a DFAM application to optimize a tooling part for robust design performing in an extremely varying operational environment for a specialized, highly renowned contract manufacturer in Germany: Ellenberger.
The evidence was to be produced for a device exposed to extreme structural loadings and strongly varying service conditions: a robotic gripper mounted onto a multi-axis robot.
The reference installation sees a 3D camera vision teaching the robot how to manipulate randomly orientated parts individually inside a bulk-container until it could pick those massive parts up. During this operation, the machinery can get caught and collisions can occur. Although the robot itself provides safety measures to prevent mechanical overloading, such an incident would have fatal consequences for a gripper designed for only one determined load-case.
What if a standard, heavy solid metal CNC’ed gripper was to be replaced for spare part maintenance – or in general – with a 3D printed part of similar function?
Rafinex and Nuform identified the potential of this gripper, which was more challenging than a simple robot end-of-arm application.
But how to effectively introduce the uncertainty without spending days on the Why’s and How’s of the setup? According to Rafinex, the embedded stochastics enabled rapid modeling and optimization of the gripper under such varying requirements.
Generally, the light-weighting of any accelerated machine components would allow increasing speed, reduce cycle times and boost the overall productivity. In addition, the reduced mass directly results in improved positioning accuracy and less energy consumption. But practically, it also means that a robust component is likely to suffer less stress peaks which benefits life cycle and/or maintenance costs. However, there is an apparent target conflict between maximizing weight reduction and ensuring robustness and uncertain load situation, which can only be addressed with advanced stochastics methods.
In the subsequent steps of the project, the applications were systematically identified. The load cases were assessed and the optimal material based on the mechanical and physical requirements were selected. Then the material properties were analyzed, taking into account the influence of the manufacturing and post-processing processes. The obtained real-world data was then used as the basis for the following design optimization. In Möbius, the various load cases were simulated and validated, including variations of the force direction by means of stochastics.
Stochastic load optimizations were initiated by taking into account deviations of the force direction from the ideal case and by limiting the degrees of freedom within the design space in foresight of the printing process. As such, overhangs were to be avoided. In addition, a pre-compensation of deformations was achieved to improve beyond the reference system's status quo.
As a result, Möbius digital output is a high fidelity, high surface quality mesh exported via STL or Nastran and directly usable to generate Gcode. The optimized gripper design was then printed on an EOS M 290 machine via DMLS with some limited post-processing of sections that needed highest precision.
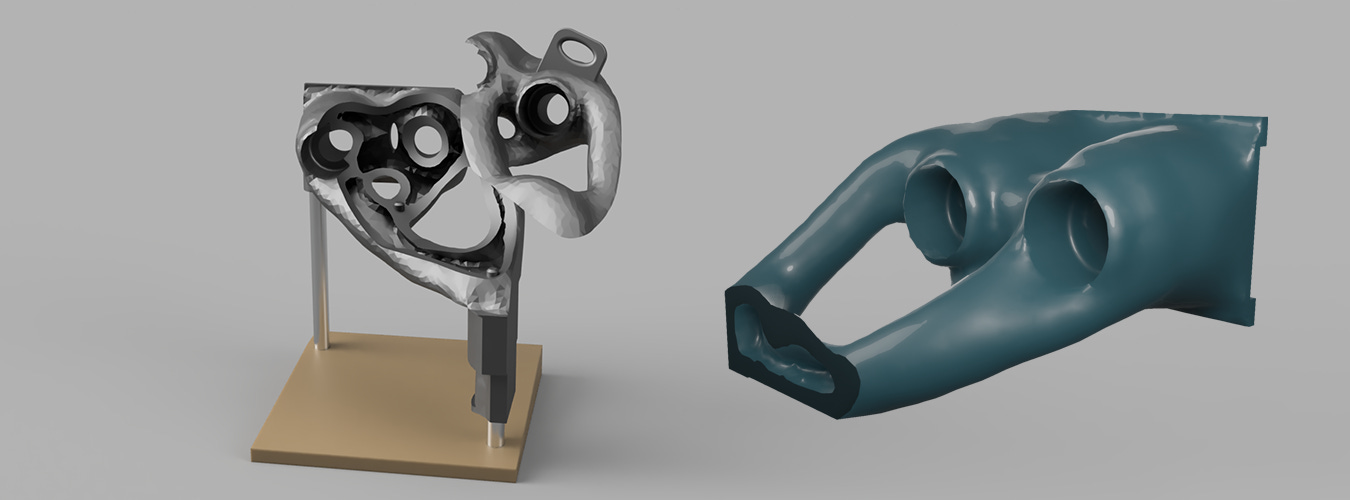
Möbius produces a design which anticipates the effect of this wide variety of relevant load paths. Moreover, the design is functional and shows a plausible, efficient mass distribution for functional purposes mainly driven by stiffness.
Interestingly – and uniquely at the same time - the optimized gripper is hollow, and its stiffness is achieved via the walls of the topology! The project members confirm that the DFAM application produced a superior design in all aspects compared to the reference.
So, in essence, it should CERTAINLY be worth discussing whether DFAM - as much as all other solutions for engineering design – need to include effects of UNCERTAINTY for designs.
The Gripper project gives evidence that optimizing a design for AM with stochastics can actually do the squaring of the circle: to produce an AM compatible, robust design which is fit for industrial use with less weight and improved performance.
To learn more about Möbius Stochastic Topology Optimizer contact Rafinex for a trial.
Reach out if you have a DfAM case study you would like to share.